Supply Chain Attack Detection
Traditional SCA solutions scan your code for vulnerabilities but miss the mark on software supply chain attacks through 3rd-party code, such as breached upstream packages and compromised build processes.
Malicious code injection attacks – as seen in the well-known 2020 SolarWinds attack – are a highly dangerous, modern attack vector that poses an imminent threat to you and your customers. Myrror’s Binary-To-Source Analysis mechanism inside our Software Integrity AI Engine detects attacks across your entire supply chain, including build pipelines – making sure no malicious code ever makes in into production.
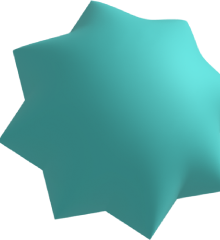
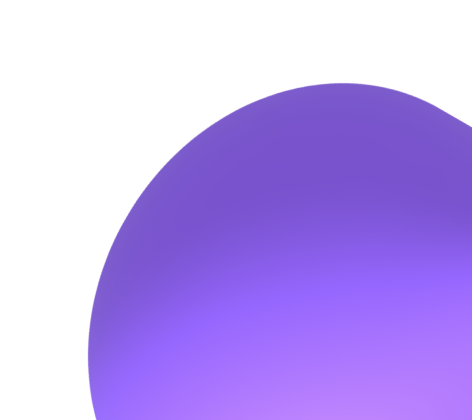
Vulnerabilities VS. Supply Chain Attacks
Supply chain attacks are an entirely different beast compared to traditional vulnerabilities, with characteristics that make them harder to defend against.
A supply chain attack:
- It is a deliberate malicious activity, unlike a vulnerability, which is more often than not unintentional and the result of a non-deliberate mistake
- Lacks specific CVE identification
- Untracked by standard SCAs and public databases
-
Typically, it has already been attempted to be exploited by the time you discover it
Software Integrity AI Engine
Myrror’s Software Integrity AI Engine detects mismatches between the source code and its corresponding binary artifacts. Utilizing Binary-To-Source Static Analysis and AI validation, it ensures all discrepancies are accounted for. This empowers AppSec personnel and developers to identify supply chain attacks hidden in plain sight—before reaching production.
Protect Your Build Process
Myrror can detect supply chain attacks before they affect all of the environments in which your code is being ran, including Dev, QA and Staging.
Additionally, it ensures no vulnerable dependencies are used in the CI/CD pipeline, containing potential damage and catching the threat earlier in the process.

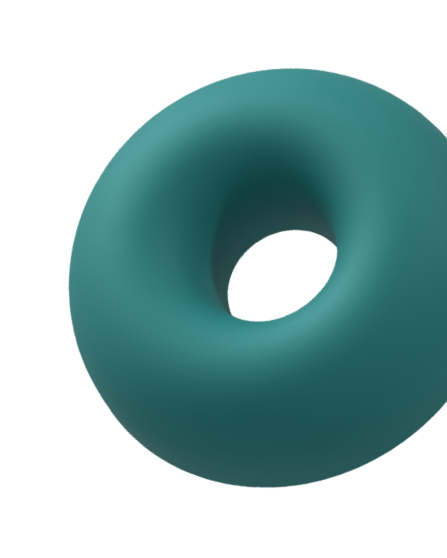
How It Works
Step 1
Myrror’s technology decompiles your binaries back into the source code, including all the direct and transitive dependencies within the applications.
Step 2
Our machine learning models compare the source code you wrote to the version generated from your binaries, looking for unexpected strings in the compiled version.